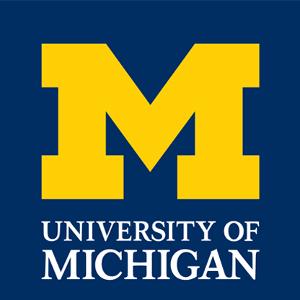
UM Hydrogen Absorbents
Project ID | 265cf506-6bc5-48d7-98a8-d6deedc8206c |
---|
Optimized Hydrogen Adsorbents via Machine Learning and Crystal Engineering
Recipient University of Michigan/UM (PI: Don Siegel)
Abstract This project aims to overcome volumetric limitations associated with physisorptive hydrogen storage at both the materials and system level. This goal will be achieved using a combination of computational techniques and experimental synthesis and testing. Our efforts will target storage media based on metal-organic frameworks (MOFs), a class of hydrogen adsorbents with highly tunable properties. At the materials level, machine learning methods will be applied to our database of 476,007 real and hypothetical MOFs. This analysis will guide the discovery of new compounds that can break through the so-called Volumetric Ceiling. This performance ceiling was identified in our prior screening studies; it reveals that no known MOFs can surpass a usable volumetric capacity of 40 g H2/L (assuming an isothermal pressure swing between 100 and 5 bar at 77 K). In contrast to the conventional approach to MOF discovery, where capacity is predicted from a known crystal structure, this project aims to invert this process and ‘reverse engineer’ optimal MOFs with the aid of machine learning. The most promising compounds will be synthesized and assessed experimentally with respect to their usable hydrogen capacities. At the system level, we will develop crystal growth and processing techniques that result in MOF-based adsorbent beds with low void fractions. Packing inefficiencies have the potential to negate improvements in volumetric performance achieved at the materials level. This project aims to close this performance gap by developing synthetic procedures that optimize particle morphology and size distribution.